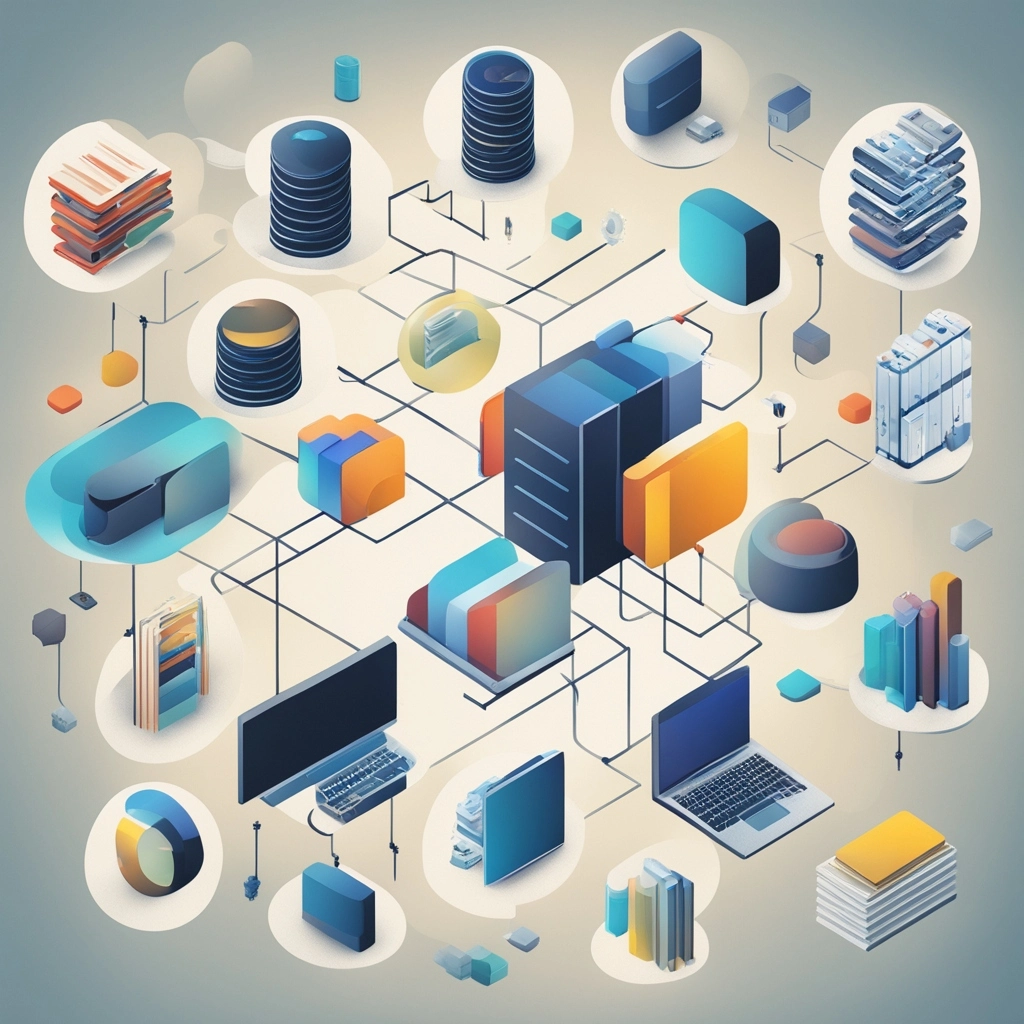
Expenditure data mapping
Impact evaluation is one of the most widespread practices in quantitative social sciences. Invariably, it requires data on interventions and outcomes. Despite its generalised adoption, impact evaluation is limited to a reduced number of policy domains where data are available. Ironically, in many countries, there exist government expenditure data across thousands of policies that are not linked to relevant outcome variables. Manually mapping expenditure into indicators can be prohibitively expensive to many governments, severely limiting their ability to integrate impact evaluation in the policy cycle. This research investigates different computational approaches to alleviate the expenditure-outcome linkage problem by provideing automated mapping tools.
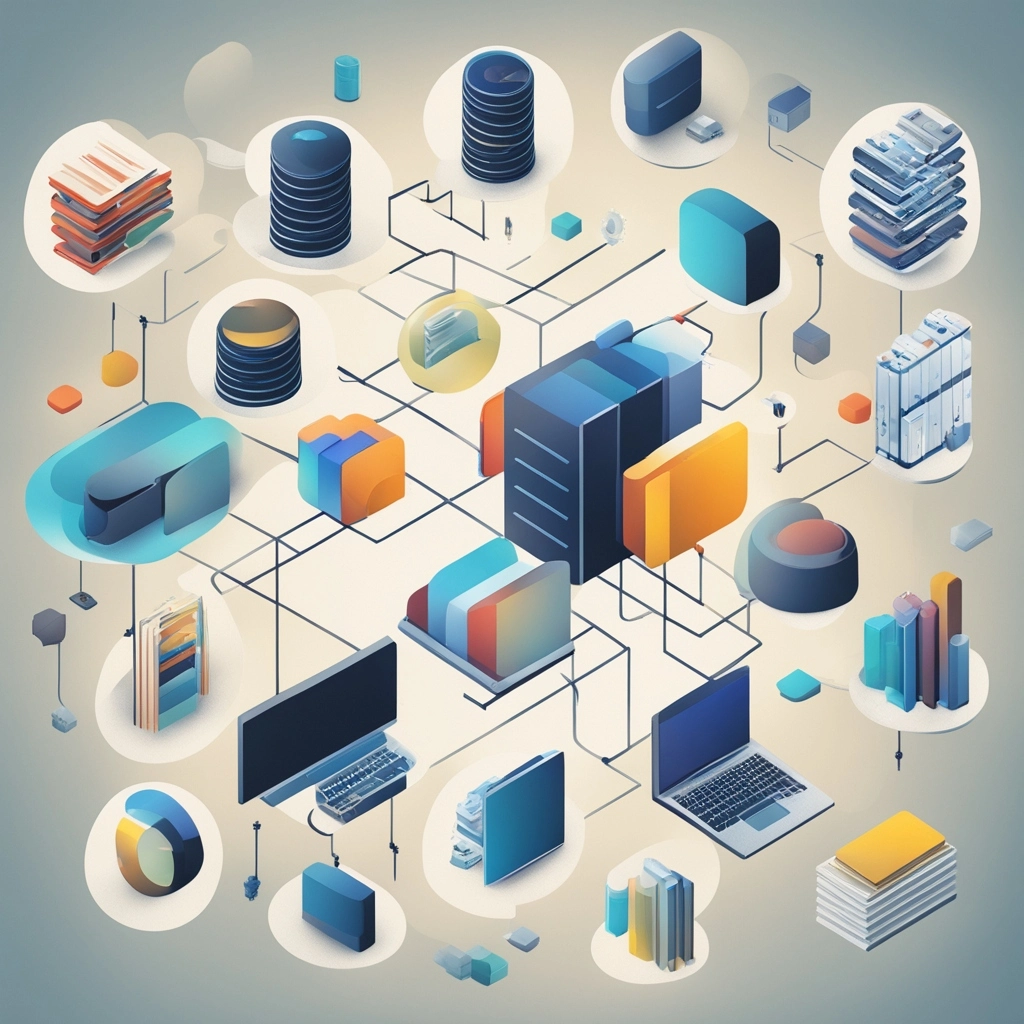
Automatic SDG budget tagging
Published on: 2023Despite the wide spread use of impact evaluation techniques, data that links detailed budget lines to outcome indicators is surprisingly scant. Performing this mapping manually can be prohibitively expensive for many countries. The idea behind this paper was to test if natural language processing could provide a reliable tool for mapping budgets into indicators automatically.
Generalized LLMs Framework to Support Public Health Financing
Published on: 2024Our initial work using NLP to link short-text budget lines and outcome indicators showed promises and limits; for example, difficulties in correctly classifying data from country A when trained with information from country B. Here, we use large-language models to overcome such limitations and develop a model-agnostic framework to generate better mappings.